PERSPECTIVE: How Predictive AI Will Change Cybersecurity in 2021
- Jenny Christiansen
- Nov 1, 2021
- 5 min read
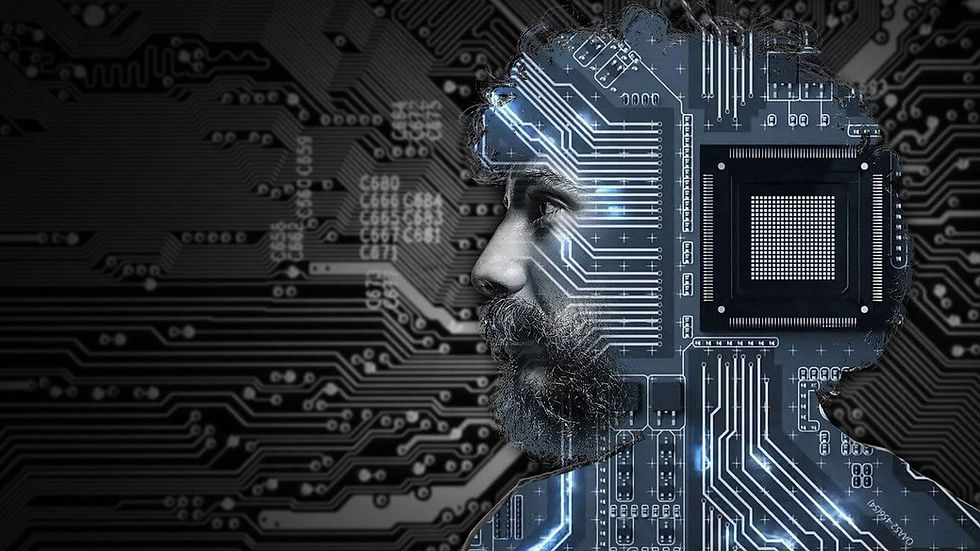
AI-enhanced cybersecurity is a must in 2021 and beyond. Clearly, the industry agrees – you’ll find an endless list of AI security platforms in the marketplace. What do vendors really mean when they use the term “artificial intelligence?” AI can be a fluid term, and sometimes mean different things to different people, and although marketing teams at cyber companies are using this ambiguity to their advantage, too often when it comes to the actual implementation and use of these platforms, the technology and promise fall short of AI in its true scientific sense.
But this isn’t always the case. Some artificial intelligence is and will be groundbreaking for the cybersecurity industry. For example, predictive “Third-Wave AI,” which is a term originally coined by DARPA to mean contextual and self-adaptable without the need for human training and tuning, can empower organizations to shut down threats before they happen, free from the restrictions and encumbrances of rules-based platforms like SIEM and other legacy AI-enhanced options.
Before you invest in a cybersecurity platform upgrade, carefully consider your options. Second-wave AI solutions may work in the short term, but modern cyber criminals have devised countless ways to break these platforms and programs. To fend off data breaches, malware, ransom attacks and other cyber crimes, SOCs will need more robust, third-wave AI solutions.
What is Third-Wave AI?
Predictive AI has been a part of cybersecurity for several years now, to varying degrees. The biggest distinction between legacy solutions and modern AI is that third-wave, predictive AI detects and surfaces threats in real time.
The U.S. Defense Advanced Research Projects Agency (DARPA) outlines three eras of AI:
First-wave, rules-based AI enabled “reasoning over narrowly defined problems” with a reduced level of certainty, like early computer chess matches or tax prep software.
Second-wave, or machine-learning AI, is based on “training statistical models on big data,” with minimal capacity for reasoning.
Third-wave, or unsupervised-learning AI, is context-aware. Machines with third-wave AI “adapt to changing situations.”
Predictive AI is a type of machine learning that automatically collects, analyzes and tests data. As it relates to cybersecurity, this technology is often seen in applications like anomaly detection platforms, threat detection and cybercrime prevention.
Predictive AI is patterned on the human brain but powered by the immense power and speed made possible only through computing processes. Today’s strongest systems are powered by quantum computing.
What’s Wrong with Second-Wave AI?
Until fairly recently, enterprises and medium-size organizations tended to work with traditional cybersecurity platforms based on first- and second-wave AI. One particularly popular choice has been SIEM (Security Information and Event Management) systems, which rely on a set of rules that “train” AI to detect network anomalies based on expected behavior.
SIEM looks promising on paper, but as many organizations soon became aware, the approach is fundamentally flawed. One overarching issue is the ongoing costs created by SIEM. Basic log storage, incremental analytics and maintenance are all quite costly (and unavoidable).
Security analyst talent is often wasted by SIEM platform functions, as well, due to an overabundance of false positives created in response to context limitations. There are only so many rules the human team can create, and since modern networks rely on constantly evolving baseline behavior, it would be impossible to keep up with all the necessary rules, anyway.
How Predictive AI Bolsters Network Security
Predictive AI can power modern, responsive cybersecurity platforms, outperforming previous-generation solutions in several key areas.
Data Overload
Because third-wave AI-enabled security monitoring detects and surfaces threats in real time, before they can compromise your network, there’s no need to accumulate and store massive amounts of data. Best-in-class AI can identify patterns and develop a humanlike understanding of what normal traffic looks like, even within constantly changing conditions.
Approach to Expected Baseline Network Activity
Free from human tuning, self-supervised (third-wave) AI learns over time how to identify and fix issues that traditional solutions can’t solve. When there is a deviation from expected baseline behavior, predictive AI quickly finds it and alerts security.
Rules-based SIEM platforms operate on a similar principle – detecting anomalous behavior by comparing activity to expected behavior. In the real world, any SOC will likely attest that “expected” behavior can change on a dime.
For example, when the world’s workforce abruptly shifted to work-from-home models en masse, any notion of “expected” or “normal” went right out the window. Millions of new, remote connections, all at once, were certainly unexpected by most security platforms, but these connections weren’t really abnormal.
Associated behaviors were not actually anomalous. Still, security analysts working for organizations relying on SIEM faced a growing mountain of false positives they had to sort through. In the meantime, cyber criminals who had been waiting for a moment like this for years swooped right in. Not only did bad actors seek out network vulnerabilities opened up by these SIEM and similar issues, but they wasted no time unleashing phishing schemes while they knew security teams would be busy addressing immediate network issues.
On the flip side, organizations that had invested in third-wave AI solutions experienced far fewer issues. These systems create an evolving baseline of normal network behavior. As a “new normal” took hold for these organizations, their third-wave AI solutions were able to adjust on the fly.
Zero Day Attack Capabilities
Zero day attacks like the Solarwinds attack on U.S. federal agencies, which made headlines at the end of 2020, can be devastating to an organization. Within minutes, an entire network can become compromised, after hackers have been inside the network for months or years, completely undetected.
Third-wave AI helps to stave off zero day attacks the instant bad actors make their move. Real-time threat detection means just that. In a rules-based system, there’s a much higher risk of losing precious response time. By the time a security analyst figures out what’s happening, the damage may well be done.
Looking Ahead: Predictive AI in 2021… and Beyond
To keep ahead of the current crop (and tomorrow’s crop) of tech-savvy cybercriminals, organizations will need to invest in cybersecurity solutions that are streamlined, powerful and powered by predictive AI.
For too long, modern SOCs have dumped millions of dollars into solutions that are failing at increasing rates. Enterprises and organizations of every size are losing revenue, constantly dealing with the financial and operational impacts of data loss while failing to address the fundamental issues with their security solutions, all the same.
Unsupervised, predictive AI is the best path forward for modern SOCs. These systems offer a centralized solution that addresses the functional requirements of anomaly detection platforms, SIEM and UBA with the added benefits of predictive, self-learning AI. Third-wave AI delivers true, real-time protection for networks assets on-prem, in the cloud, and across connected devices.
The views expressed here are the writer’s and are not necessarily endorsed by Homeland Security Today, which welcomes a broad range of viewpoints in support of securing our homeland. To submit a piece for consideration, email editor@hstoday.us. Our editorial guidelines can be found here.