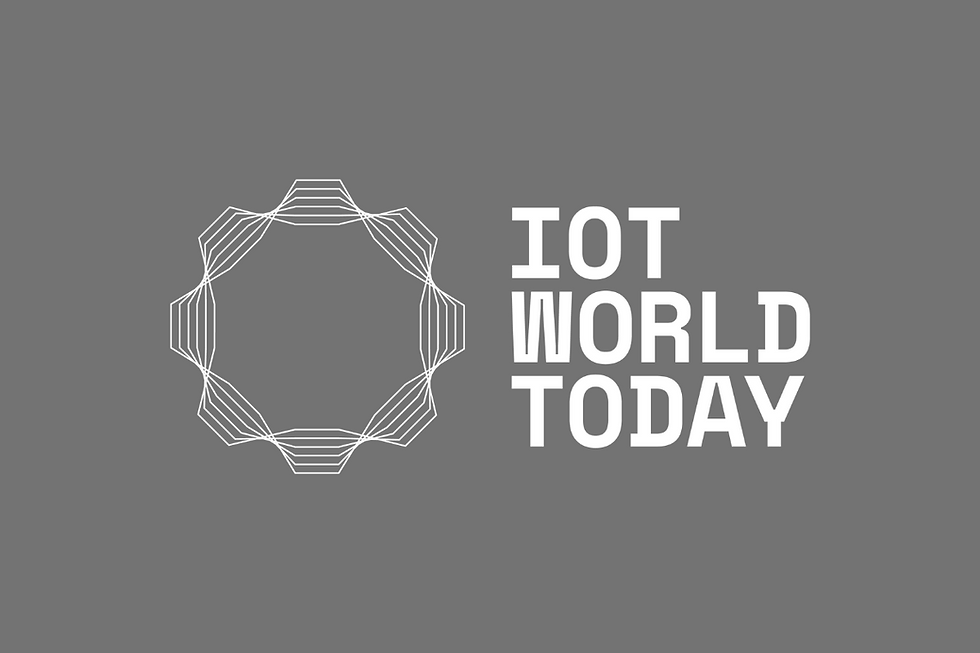
Quantum-powered AI has been shown to perform better in image classification and applying combinatorial reasoning optimization to chatbots.
Enrique Solano, Founder, Kipu Quantum
February 3, 2025 - IoT World Today
There has been a great deal of interest in the AI industry in how AI tools might help quantum computing develop. However, the relationship between AI and quantum computing goes both ways. Yet there has been relatively little discussion of how quantum algorithms could help solve some of the problems that face AI tools.
There has also been a great deal of discussion among corporate and institutional players about the received wisdom that quantum computers will only deliver added value once a fault-tolerant quantum computer delivers usefulness for an industry application, something which it is anticipated will not happen for at least five to 10 years. However, in private, quantum startups are fiercely competing to achieve quantum advantage within the next few months.
Indeed, in the AI sphere, quantum advantage has already been reached. Hardware-specific quantum algorithms applied to AI have been shown to have outperformed traditional AI tools in the areas of image classification in health care and generative AI used in chatbots.
It has also been widely and routinely said that the creation of a quantum computer that can outperform classical computers has yet to be achieved. As expert readers will be aware, this is not true, it’s simply a commercial position that has been adopted by many people. Indeed, last December Google announced – in findings published by Nature – that it had passed an important milestone in overcoming the inherent instability of quantum systems, achieving a level of quantum error correction below the surface code threshold. In the same article, Google described how it ran a quantum algorithm that cannot be reproduced by any classical supercomputer unless it runs for a time longer than the life of the universe.
This development was cautiously reported as the passing of a major hurdle in building quantum computers and a sign that the first full-scale quantum computer systems might be operational by the end of the decade, i.e. in five to six years. Actually, functional quantum computers do exist and companies will – in a timescale much shorter than five years – be able to use quantum algorithms that can solve granular problems in many industries. These problems notably include combinatorial optimization, a hardcore optimization problem that underlies chemistry, finance and many life science applications and which involves a level of complexity that is a truly unsolvable problem for classic supercomputing centers because they are unable to progress beyond a certain number of variables.
The immediate industrial applications of combinatorial optimization include portfolio optimization and asset prediction in the finance industry, protein folding and robot arm sequencing in the life sciences industry and signal re-routing in the telecoms section. There are also very important applications for the AI industry.
The first area where quantum advantage has been reached for AI tools is image classification. We recently published a peer-reviewed paper that a quantum algorithm can better predict breast cancer than traditional AI. This is not due to its speed – usually the main selling point of quantum computing – but because quantum algorithms are extremely good at detecting defects in images. This means that the breast cancer-detecting algorithm needs 1,000 times fewer images than an AI detection tool to accurately predict a cancer and produces more accurate results.
This reduced demand for images has a knock-on effect on health care outcomes, as fewer images mean a reduced amount of radiation to which the body is exposed. Enhanced defect detection was achieved through the use of quantum convolutional neural networks, an application of neural networks combined with quantum computers which has the potential not only for health care but also for navigation systems, satellite imaging and astrophysics.
Another area in which quantum computing has already demonstrated that it can outperform classic AI tools is the application of optimization combinatorial reasoning to AI chatbots. In this area, quantum computing has great potential to reduce energy consumption, which is one of the major bottlenecks faced by chatbot providers. Indeed Sam Altman said last year that he might even consider building a nuclear plant to provide the energy needed by ChatGPT However, when a quantum optimization algorithm is used for generative AI tools, the energy consumption is negligible in comparison. Quantum algorithms may also have the ability to offer enhanced speed which might facilitate the competition against DeepSeek which is currently seen to be so crucial.
These two examples of quantum advantage in AI need not be taken as explosive news. Computational quantum advantage for industry use cases is commonly presented as a very narrow line that, once crossed, will utterly transform the universe into a quantum future. However, it is not, in fact, a thin line but a very broad region where claims, counterclaims, corrections, improvements and enhancements are about to start to occur. We have already entered this region and over the next 12 to 24 months we can expect to see its impact on the AI industry materialize and solidify.